The application of multivariate and iterative testing methodologies to playable and interactive advertisement creatives allows Kaden to boost the performance of an already high-performing ad unit and maximize campaign results. We optimize at a site-level through this testing process, targeting user demographics and interests in a contextual fashion to drive site-level understandings, click-through-rates and, ultimately, installs and ROAS. For more details behind this essential aspect of our programmatic mobile operations, please read on.
What is multivariate testing?
In case you aren’t already familiar with multivariate creative testing, it is simply the application of two or more variations of one ad creative within a uniform environment to understand which variation performs best. Given that our technology applies a range of device-targeting strategies, this ‘environment’ is defined as an inventory site against an applied user segment. Okay, maybe that wasn’t so ‘simple’, so let’s break it down.
For instance, does the colour of a call-to-action button generate a tangible difference in click-through-rates? How about square icons or rounded? Which call-to-action drives the most clicks? Do users finishing their workout in a fitness app want a salad, or a pizza? Can we run a creative test to develop an understanding of that for a delivery or food app campaign? Remember that even just a 0.1% difference in CTRs can be huge when extrapolated out to ROAS.
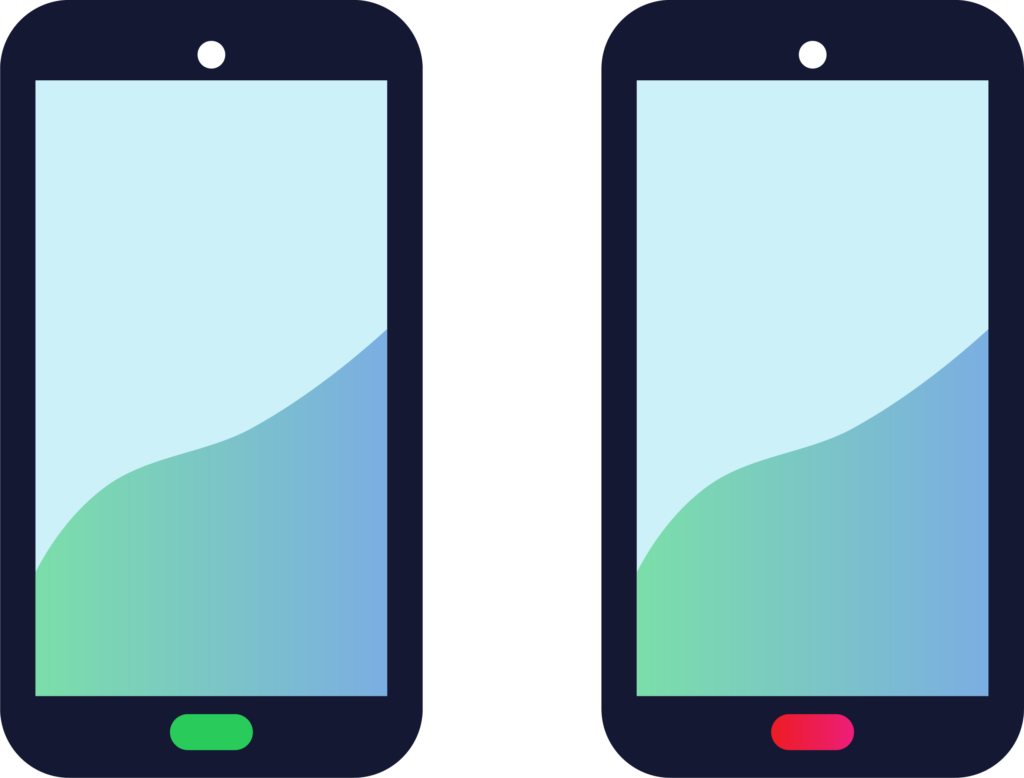
This is not a new methodology. Multivariate creative testing has been applied in display graphic advertising for many years. However, it has been difficult until now to apply this concept to playable ad units, primarily due to their high costs and associated times to produce. At Kaden, our creative services staff can individually develop 3 unique playable units and 20 iterations of each every day, from within a code-free environment. We are able to apply multiple versions of the same ad even when beginning a campaign, and can progressively and iteratively develop on those variants in a staged process. We do this while allowing our AI to apply, over time, learnings at a macro-level from experiments run at a micro, site-level.
Let’s look at some examples:
Learning to target a Latinx audience
Currently, we are running a campaign for a food delivery app expanding across South America. As they want to target a Latinx audience it makes sense for us to start by walking users through purchasing several different Latin cuisines in the interactive advertisement experience. Then, it is time to challenge that assumption. Can we run tests to determine if users actually prefer to order Asian food? Do their preferences change depending on whether they are utilising an app in English, or in Spanish or Portuguese? These and other standard tests for color (red or green “order” button), offerings (10% Off versus No Fee Delivery), and CTA (“Order Now” versus “Place Order”) allow us to gradually assess the preferences of the South American users and apply those learnings in a mechanical fashion to bring about sustainable scalability. Of course, there are market studies which can suggest these kinds of preferences ahead of time. Our Account Data Managers utilise such studies when positioning the strategic application of machines to the problem at the beginning of a campaign, though there is nothing is better, in our view, than cold, hard CTR, CVR, and ROAS numbers.
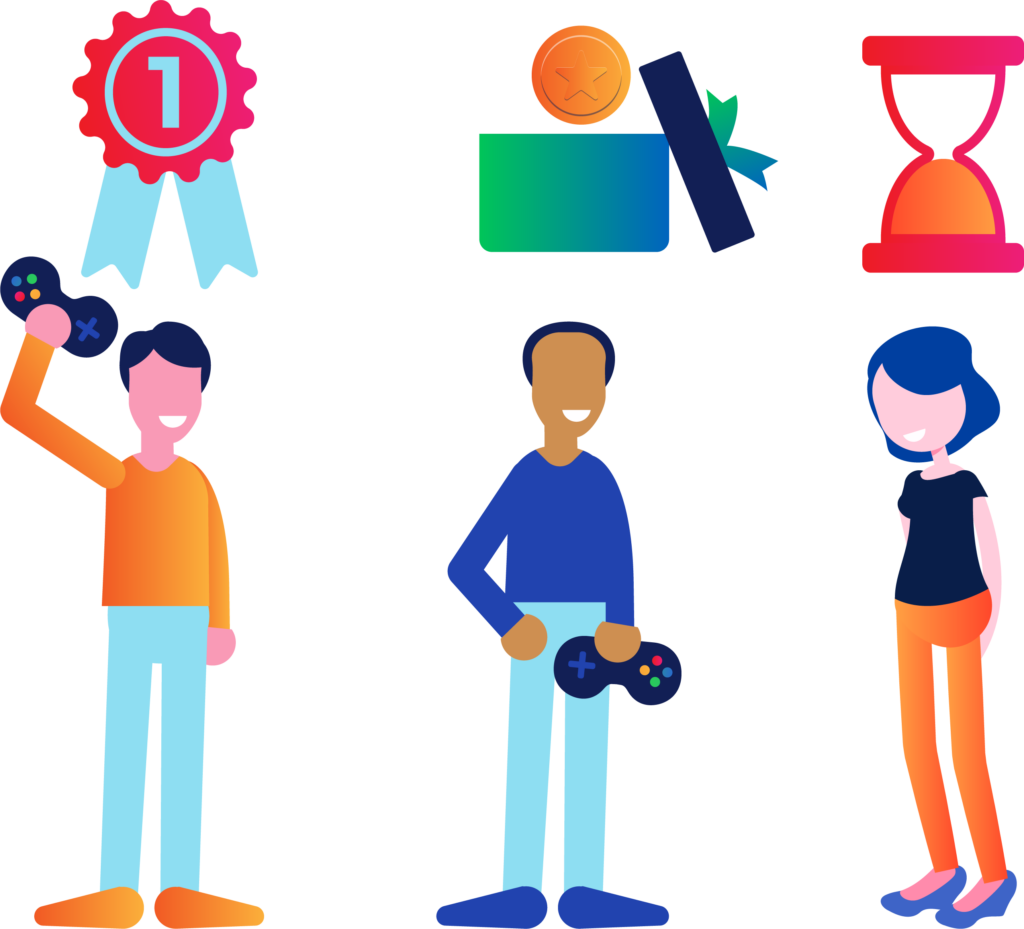
Gaming-specific considerations
Gaming is often the first vertical that comes to mind when discussing playables, even when it is not the only vertical that can implement this strategy effectively. There are key considerations for gaming, and some cool things that we think can be done when matching playables to multivariate strategies for gaming advertisers.
We might, for instance, and depending on the game at hand, want to define particular user groups and vary the ads being shown to each. There could be competitive players, who will do anything to level up or outrank other players. There could be collection gamers, who want to complete as much of the game as possible (which can mean not only beating every level, but winning every trophy and unlocking every weapon, for example). There could also be casual gamers, who need an enticing reason to engage with a game every day or they may move on to another. At a site-level, it is common that these different groups of players reside in pools of users (one of the reasons why a flexible bid strategy is required at a site-level!). That provides us with a great opportunity: to consider “how we entice competitive gamers in apps that competitive gamers use?”…
Well, we could, for instance, test playable variations that walks the user through applying a skill tree, or through a fight-out, giving them a taste of what it would be like to be a top ranked player within the game. For gamers who collect or prefer aesthetic upgrades, we might offer an experience that has them customise a character, or apply a clothing set that provides an extra boost. Only once we discover what type of playable experience performs best on any given particular site do we start to make iterative changes, so ensuring that we apply a mix of playable units when beginning the campaign is key.
One more benefit: avoid ad fatigue
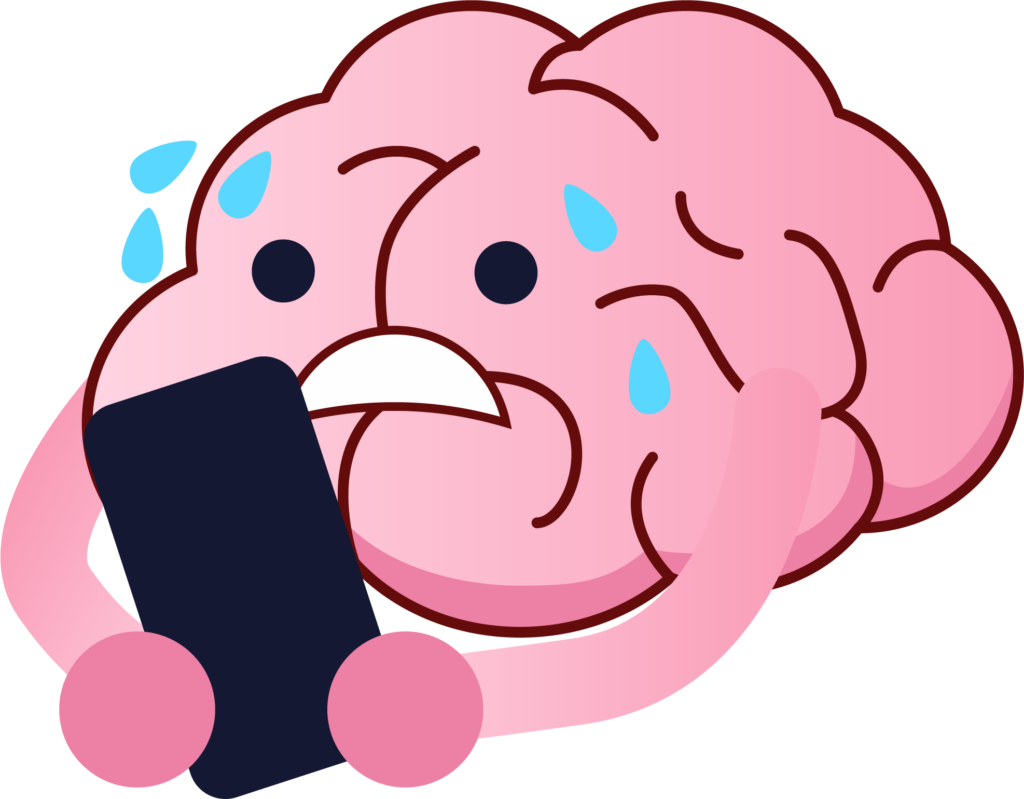
One forgotten benefit of multivariate and iterative testing – alongside the more novel characteristics of playable creatives – is that they help your campaigns to avoid ad fatigue. This is possible because while a user may see your ad more than once, if the ad is slightly different the brain cannot completely ignore it. It is a survival mechanism. In fact, humans are naturally gifted at learning an experience over time so that we can deprioritise it as a short-term memory curiosity (this is called ‘spaced-repetitive learning’), which is what causes diminishing returns for many advertisement campaigns. Multiple variations on the same topic can help to break a user out of this memory feedback loop by forcing them to consider that there is something new to be learned about the topic at hand.
Why Kaden
At Kaden, we combine machine learning and the human insight of our Account Data Managers to help determine the most effective iterations in each set. You now know how we can improve on an already engaging format (playables) with high tech performance-boosting methods (multivariate testing). Powered by AI, machine learning, and human experience, we can build the most effective campaigns to get your app the results you want. Together, let’s find your ideal users.
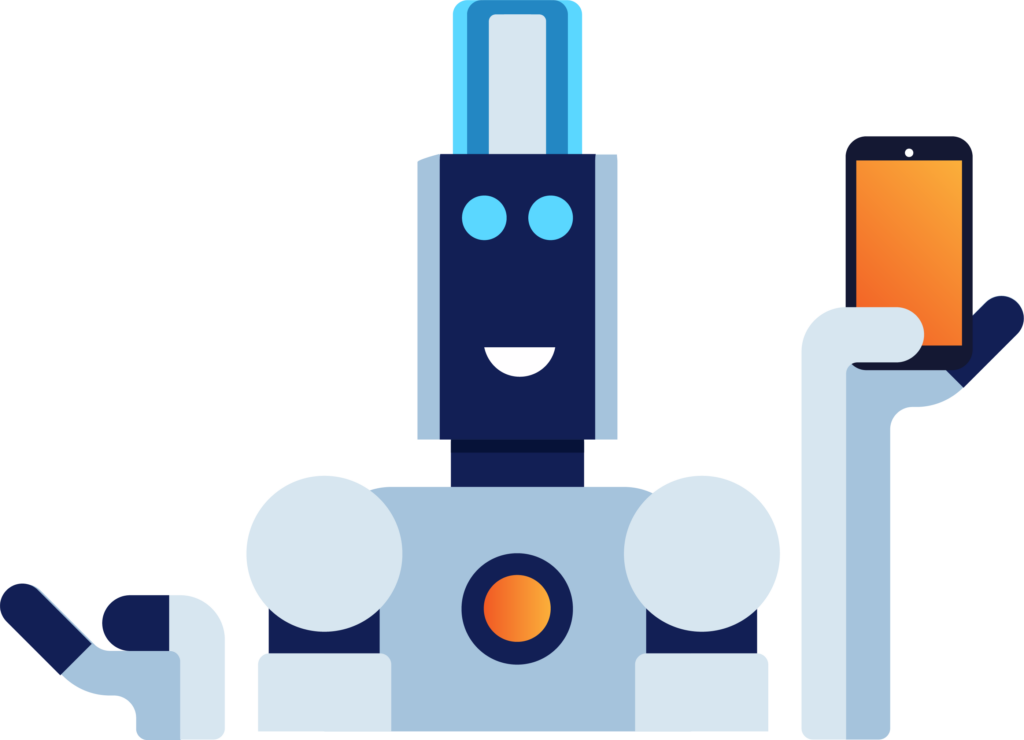